Heading
Emerging GenAI Middleware Architecture
Emerging GenAI Middleware Architecture
Being in a volatile technological era, middleware projects confront loads of factors that can determine how effective they are. Despite the latest developments in contemporary middleware, complications that come with integration still persist, thereby demanding careful planning and expertise in enterprise data management as well as a deep understanding of business processes and middleware platforms. In over sixty years, organizations have been challenged by integration barriers where various schedules and costs have led to their smooth realization.
GenAI is positioned as the next phase of evolution in middleware architecture that has unprecedented power to change how applications and systems interact within an IT ecosystem of an organization. Companies are able to achieve fast, cheap and faultless content analysis, seamless integration, task automation, as well as application updates through utilizing the strength of GenAI.
The question still remains: How can GenAI master these complex challenges thus redefining the future of middleware architecture?
Let us consider how GenAI IT support emerging as a trend in middleware architecture in this blog.
Understanding GenAI
In recent years, the integration of sophisticated computer skills with artificial intelligence has resulted in GenAI (Generative Artificial Intelligence). GenAI is a new paradigm which has surfaced as a result of advancement in these two areas. It signifies the end result of several decades’ worth of AI research and development, whose aim is to build intelligent systems with generative capabilities that are similar to those of human beings.
Definition and Concept
GenAI can be described as a category of artificial intelligence systems that show flexible intellectual capacities which allow them to see, think, understand, learn, and change in various tasks and circumstances. In contrast to narrow AI systems built for specific purposes, GenAI aims to develop a sort of universal intelligence that can transfer the knowledge and skills across different domains.
A key idea behind GenAI is generative intelligence. It means the ability of an AI system to use learned knowledge and skills in new situations or tasks it has never seen before. This property makes GenAI capable of handling complex problems and adapting to changing environments that are perfect for numerous practical applications.
Key Components and Technologies
To attain generative intelligence, GenAI service desk systems employ various technologies and methodologies. Some of the main parts and methods are as follows:
1. Machine Learning
Machine learning algorithms enable GenAI systems to learn from data and enhance their performance over time. Deep learning, reinforcement learning, and evolutionary algorithms are some techniques that have a significant impact on training intelligent models.
2. Natural Language Processing (NLP)
NLP helps GenAI understand human language for communication with users. Advanced NLP models, including transformers and recurrent neural networks, empower GenAI to understand text data context, sentiment, and semantics.
3. Computer Vision
Through computer vision technology GenAI perceive and interpret visual information derived from images or videos. For example, CNNs (convolutional neural networks), which are deep learning architectures, enable GenAI to recognize objects, scenes, and patterns in visual data with extremely high levels of accuracy.
4. Knowledge Representation
To represent knowledge, GenAI systems use elaborate techniques. Knowledge graphs similar to ontologies or semantic networks create structured frameworks for organizing and processing information that allow deciding or inferring connections between entities by Gen AI.
Applications in Various Industries
There are several ways in which GenAI can be utilized to transform different sectors and industries by enabling smart solutions for complex problems. Some of the important uses include:
1. Healthcare
GenAI-powered service systems might aid healthcare experts in diagnosing diseases, predicting patient outcomes, and developing customized treatment plans according to individual patients’ records.
2. Finance
In finance, GenAI algorithms are used for the detection of frauds, risk assessment, algorithmic trading, and customer service automation.
3. Transportation
This technology helps autonomous cars navigate safely and with efficiency reducing traffic jam, accidents and making transportation sustainable.
4. Education
As far as personalized learning is concerned; GenAI tools assist in tailoring education content based on student’s unique needs and preferences,
5. Manufacturing
Provided that there is adequate Artificial intelligence support in the manufacturing system, it will improve productivity through process improvements such as optimizing production schedules, predicting equipment failure rates, and enhancing quality control, among others.
To unleash its full potential in addressing social challenges and facilitating innovations across diverse domains, an understanding of GenAI capabilities and its applications is vital.
Emergence of GenAI in Middleware Architecture
Middleware architecture is a crucial layer that enables communication and integration between incompatible systems and applications in distributed computing environments. In the past, middleware solutions focused on offering fundamental messaging, data management and transaction processing features. However, the advent of newer technologies like Generative Artificial Intelligence (GenAI) has caused a shift in the middleware architecture towards intelligence-driven solutions.
1. Evolution of Middleware Architecture
The evolution of middleware architecture has moved from simple message-passing systems to more complex platforms that can handle intricate interactions and workflows. Early middleware solutions aimed to ensure heterogeneity and dependability across various systems. As computing environments became more dynamic and diverse, middleware evolved to address scalability, performance and security issues.
2. Integration of GenAI Technologies
Another dimension of middleware architecture has been opened up by the advent of GenAI technologies, with intelligent decision-making, autonomous operation, and adaptive behavior becoming possible in distributed systems. Traditional middleware functionalities are enhanced through machine learning, natural language processing and other AI techniques by GenAI middleware which also provides for such advanced capabilities like:
a. Intelligent Data Processing
In real-time, this middleware analyzes large volumes of data to extract valuable insights and patterns that can be used in decision-making. By using the context clues and user preferences based on which it processes or prioritizes data dynamically through ML algorithms.
b. Adaptive Resource Management
It is responsible for distributing resources as well as workflow orchestration for efficiency purposes in distributed computing environments. Based on system metrics coupled with user interactions, this middle ware is able to intelligently distribute computing resources, distribute workload evenly among nodes and address any performance bottlenecks to ensure smooth running.
c. Enhanced Communication Protocols
GenAI middleware improves communication protocols by integrating natural language understanding, semantic interoperability and context-aware messaging. By incorporating natural language processing abilities, GenAI middleware allows for smooth communication and collaboration between human beings and intelligent systems which facilitate more intuitive interactions and reduce barriers to communication.
Features of Emerging GenAI Middleware
The introduction of Generative Artificial Intelligence (GenAI) into middleware architecture brings about a new breed of smart middleware solutions that possess improved communication, data processing and resource management skills in distributed computing environments.
Some distinguishing characteristics of these new-generation platforms include:
1. Intelligent Data Processing
Data that is intelligent is what GenAI middleware can do by combining machine learning algorithms with natural language processing techniques. This ensures that such middleware can analyze and grasp large amounts of data real-time, thus making them very useful for detecting patterns or extracting valuable insights in support of various applications and use cases. In this regard, GenAI middleware employs intelligent data processing to respond dynamically to changing conditions and requirements, resulting in heightened performance and productivity.
2. Resource Management that Adapts
The key distinguishing quality of GenAI middleware is its provision of adaptive resource management in distributed computing environments. To this effect, it continually tracks system metrics, user interactions, and workload patterns to distribute resources dynamically, balance workloads, and optimize performance. This is achieved by effectively managing computing resources, thus promoting efficient use of resources, scalability, and resilience even in highly dynamic, unpredictable environments.
3. Communication Protocols That Are Improved
Natural language understanding, semantic interoperability and context-aware messaging are some ways through which GenAI middleware improves communication protocols. As opposed to traditional middleware solutions that depend on inflexible communication protocols and data formats, GenAI middleware can comprehend and handle natural language inputs, thus allowing for a more intuitive human-intelligent systems communication process. Consequently, this enhanced communication capability enables seamless collaboration across distributed computing environments in sharing information as well as decision-making.
4. Autonomous Decision Making
Another remarkable characteristic of GenAI middleware is that it can make autonomous decisions depending on the learned knowledge and contextual information. This middleware applies machine learning algorithms to study the data, learn from past experiences and decide how to achieve set goals before moving. Thus, this enables the middleware to be adaptive, handle unpredictable events and still maintain its peak performance despite operating under different conditions without necessarily needing a human being for control or manual intervention.
5. Scalability and Extensibility
GenAI middleware is highly scalable and extensible: this means that it can be adjusted rapidly to meet changing requirements while providing seamless expansion of capacities so that they can hold more workloads as well as respond to increasing user requests. Typically, these architectures are modular and flexible enough, allowing developers to easily add new functionality, algorithms, or components into them.
So with such flexibility in place, GenAI middleware has been built in such a way that it can support any kind of application irrespective of its size, right from small-scale systems up to middle-ware used in organizations with thousands or millions of transactions per day at any given time without compromising the reliability as well as performance.
6. Security and Privacy
The GenAI middleware design must take into account security and privacy since intelligent systems deal with sensitive data and operations. In order to ensure availability, integrity, and confidentiality of the data, GenAI middleware has strong security mechanisms in place along with encryption methods and access controls that protect sensitive information. GenAI middleware platforms are designed to maintain privacy at all costs; therefore, they employ techniques like Federated Learning and Differential Privacy, which minimize the chances of data sharing and processing associated risks.
7. Continuous Learning and Improvement
GenAI middleware supports continuous learning and improvement using feedback loops, adaptive algorithms, self-monitoring mechanisms, etc. By learning from past experiences, user interactions as well as environmental feedbacks, Gen AI systems tend to improve performance levels by enhancing decisions made by them or adapting accordingly in accordance with changing environmental conditions over time.
The ability for a constant development is important for a GenAI service desk system to remain relevant in an ever-changing computing environment thereby ensuring its viability as well as sustainability across many years ahead.
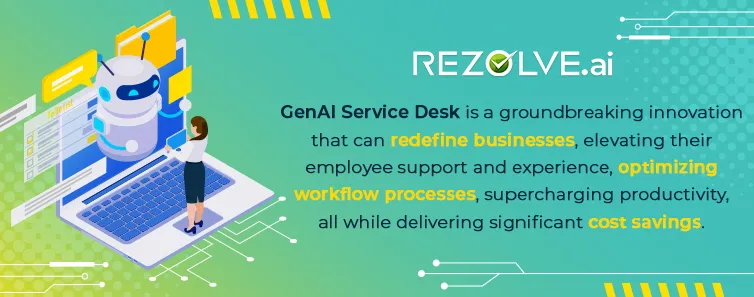
What are the Implementation Strategies?
Effective integration and deployment of distributed computing environments in Generative Artificial Intelligence (GenAI) middleware architecture has to be properly planned, designed and executed.
To effectively implement GenAI middleware solutions, several key strategies and best practices can help organizations:
Step 1 : Needs Assessment and Requirements Analysis
The organization should do a thorough needs assessment and requirements analysis before implementing GenAI middleware to identify specific use cases, business objectives, and technical requirements. On this note, it is important for all corporate stakeholders to engage users’ feedback and evaluate existing infrastructure and systems for scoping out the GenAI middleware implementation.
Step 2: Integration with Existing Systems
To ensure interoperability and compatibility, GenAI middleware needs to be integrated seamlessly with the other systems, applications or infrastructures that are already in place within organizations. Stakeholders must, therefore, take into account various aspects such as points of integration, communication protocols, or even data exchange mechanisms that will make interaction between GenAI middleware smooth about other elements within its computing environment.APIs, standards-based interfaces, or middleware connectors may assist in making this easier, thus enabling an uninterrupted data flow between numerous systems that may not be compatible.
Step 3: Development Frameworks and Tools
Selecting the right frameworks and tools for development is crucial in building and deploying GenAI middleware solutions effectively. Modern software development platforms, AI frameworks, and middleware technologies that support rapid prototyping, experimentation, and scalability should be used by organizations . Open-source frameworks such as TensorFlow, PyTorch and Apache Kafka can develop and deploy GenAI middleware applications in various computing environments.
Step 4: Model Training and Optimization
Intelligent data processing and decision-making tasks are carried out using machine learning models and algorithms with GenAI middleware. Organizations should invest time and resources in training, fine-tuning, and optimizing machine learning models to ensure accuracy, efficiency, performance. Techniques like hyperparameter tuning, model compression or transfer learning can make GenAI middleware solutions more effective as well as scalable providing them with the ability to handle large datasets or difficult computational problems.
Step 5: Dimensions of Scalability and Performance Testing
GenAI middleware must focus on scalability and performance testing, particularly in large-scale distributed computing environments. Therefore, organizations should conduct thorough tests and benchmarks to measure the scalability, throughput and latency of GenAI middleware solutions under different workloads or conditions. Some common ways of identifying bottlenecks include load testing, stress testing, and performance profiling among others that would optimize resource utilization while ensuring that GenAI middleware is reliable in production.
Step 6: Security and Compliance Issues
Taking into account the nature of data managed by intelligent systems, security and compliance are very important in implementing GenAI middleware. To avoid any breaches or unnecessary risks it is fundamental for firms to put proper security measures such as encryption methodologies, access controls among others while adhering to relevant laws and regulations; Furthermore they have to maintain regular security audits, vulnerability assessments, thus uncovering as well as helping them mitigate risks associated with deployment of GenAI middleware
Step 7: Information and Skill Developments
To put it into operation, skilled personnel in AI, ML, middleware architecture and distributed computing are needed for GenAI middleware to be successful. Consequently, organizations should invest in training as well as skill development programs to ensure employees have the knowledge and ability to design, develop, install as well as maintain GenAI middleware solutions effectively. To this effect, cross-functional collaboration and knowledge sharing including continuous learning initiatives nurtures a culture of innovation and excellence towards implementing GenAI middleware.
Step 8 : Tracking plus Improving
GenAI middleware solutions need constant tracking so that they can work optimally after deployment also requiring optimization together with maintenance for reliability from time to time. For this reason, organizations should deploy strong tools for monitoring like logging mechanisms besides alerting systems that can enable them follow system metrics while detecting anomalies or troubleshooting real-time challenges. Therefore, there is a need for continuous optimization efforts comprising such activities as performance tuning or software updates which will increase the value of any GenAI middleware deployments over time.
Conclusion
Since companies are initiating the development to take in Generative Artificial Intelligence (GenAI) as the next middleware architecture, there must be a careful strategic planning. It starts with understanding integration needs and objectives holistically within existing IT landscapes that encompass various applications, systems and data types. By stating major challenges beforehand of how it will be integrated, organizations can set up GenAI to operate effectively as a middleware of the future by expressing clear integration goals.
This comprehension of requirements will thus provide the basis for leveraging on GenAI’s transformative capability towards innovation, efficiency and agility in distributed computing environments as technology advances.
Experience the future of IT support with Rezolve.ai's GenAI-powered AITSM Solution. Book a demo today
FAQs
1. What is middleware in architecture?
Middleware in architecture means a software that intervenes between different applications to enable communication and interaction. It serves as a link, which makes it possible to have a smooth incorporation as well as exchange of information between distant systems within an interconnected environment.
2. What are the types of middleware in 3 tier architecture?
In 3 tier architecture, middleware types involve presentation middleware (for instance, web servers), application middleware (for example, application servers) as well as data middleware (such as database management systems). These layers share processing activities thus improving scalability and flexibility in software.
3. What are the 4 functions of middleware systems?
The 4 functions of middleware systems include integration, communication, management and security. Middleware allows for effective communication across dissimilar applications, integrating various components of a system efficiently managed resources, ensuring safe transmission and accessibility of data throughout the network.
4. Is middleware and API the same?
Although both middleware and APIs can be used for communication between software components, their functions are distinct. Middleware is a mediator that enables applications to communicate and integrate with each other; on the other hand APIs offer set interfaces to access some functionality or data in an application or service.
